Part 2: AI Economics, Market Dynamics and Future Prospects
Part 1 here
What is the complement to a prediction?
The most important microeconomics lesson that I didn't learn during my MBA program: "All else being equal, demand for a product increases when the prices of its complements decrease. For example, if flights to Miami become cheaper, demand for hotel rooms in Miami goes up — because more people are flying to Miami and need a room."
I stumbled upon this gem in Joel Spolsky's wonderful book, and then got a deeper dive into it when I read Shapiro and Varian's book, Information Rules - which totally reshaped how I think about tech, investing, and basically how I see the world.
Subsidising, or commoditising the complement to your product is a tried and tested strategy across industries. Microsoft famously helped commoditise the PC, much to the chagrin of IBM. In my own career, I've seen Corporate Credit Cards subsidise and/or commoditise their complement - CFO tools.
The 'product' that the purveyors of AI are selling us is a 'prediction'. A complement to the product - prediction - is data. The more high-quality data an algorithm has to learn from, the better its predictions are likely to be.
In that sense, data and predictions are complementary because the value of each increases with the quantity and quality of the other. The cost to make predictions fell, its demand increased, and data was increasingly commoditised. We gave away data in return for 'free', or freemium use cases. Search engines indexed our thoughts, words, rants, and epiphanies. The likes of Google indexed over 40 million books, while "GPT-4 has learned from a variety of licensed, created, and publicly available data sources, which may include publicly available personal information." The tide is turning on this trend, however.
Sarah Silverman is suing OpenAI, Reddit and Twitter are charging heavily for their APIs, and data is getting increasingly siloed and restricted by geography. If the New York Times vs OpenAI case does reach court, it will further cement this.
This is all an unintended consequence of the success of the GPT family.
And this is also what is likely to create a strong barrier against all the many doomsday predictions - data. Model improvements rely on easy access to data, which will increasingly be held privately. How do you scrape and mine data that just isn't available to you to learn from? And rogue actors/nation states aside, how do you legally overcome increasing demands for data localisation? GDPR, CCPA, India's data privacy bill, and China's data localisation requirements are all part of this trend. This may be further reinforced by the rise of localised LLMs like Gigachat, BritGPT and the like.
The Economics of AI
Creation Costs and Value Distribution
As AI continues to evolve, we can recognise certain patterns from two distinct eras:
1. The steam vs electricity paradigm, as documented by Agarwal et al
2. The changes brought on by the internet, many of which are documented by Shapiro and Varian in Information Rules
Shapiro and Varian wrote about how the internet turned the economics of distributing information on its head, pushing down the marginal cost of distributing information, making distribution efficient and cheap.
AI now comes to the mix by attacking creation costs in the same way that the internet attacked distribution costs. With LLMs, creating text, images, video are tending to zero marginal cost. The silly graphic below, as an example, was created by Midjourney and it did not cost me anything.
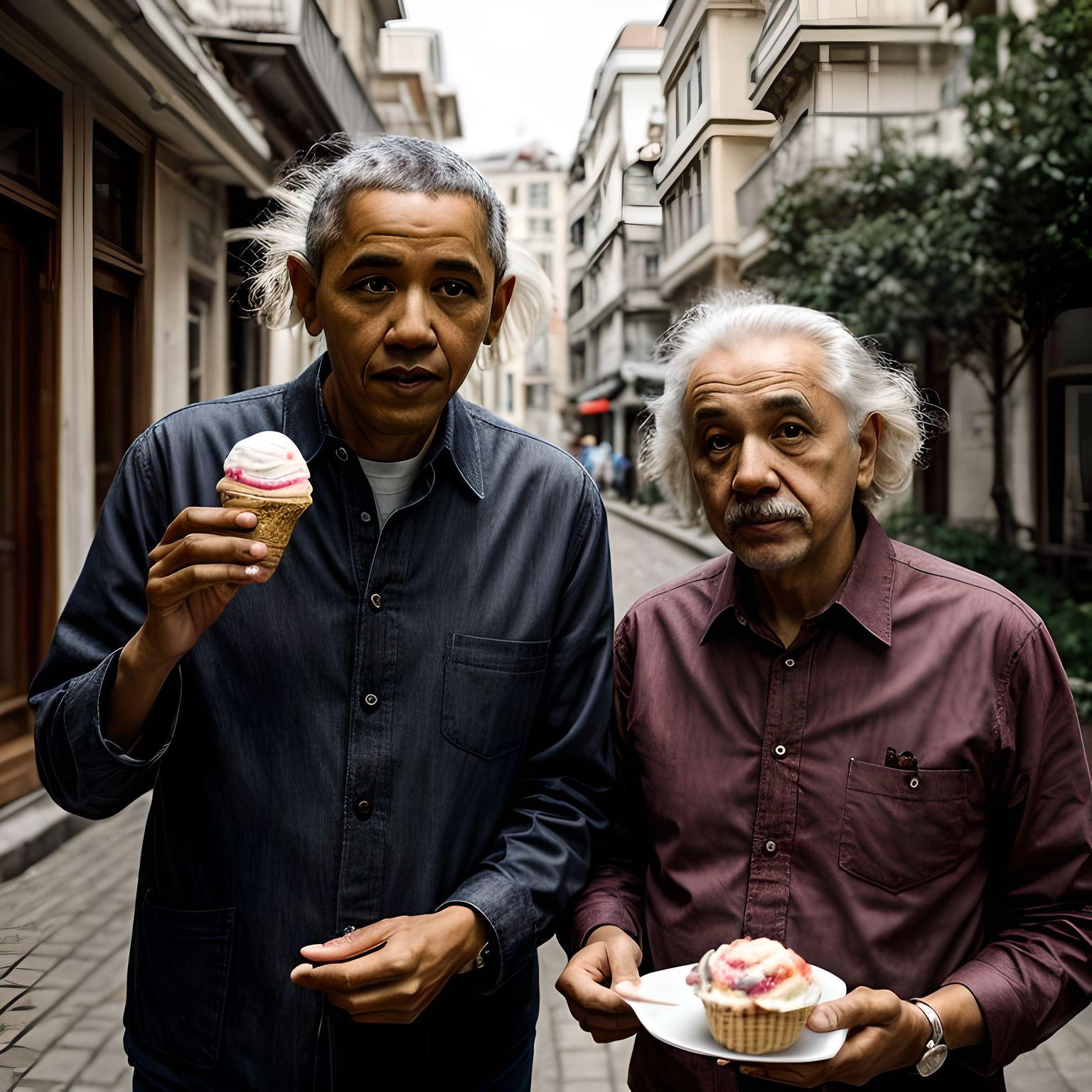
We are pushing the boundaries of traditional business models, and shaking up how economic value gets divvied up. Back in the day, a product or service's value was often linked to the cost of making it. But as the cost of creation goes down, the value of a product or service is more and more tied to its data and intellectual property.
This means that companies with a ton of data and intellectual property will be able to grab a bigger piece of the economic pie created by AI. Take Google and Facebook, for example. They've managed to grab a big chunk of the ad market by collecting and analysing user data. This data lets them target ads way more effectively, which has made them very profitable.
Much like how the internet revolutionised distribution by drastically reducing costs, AI is poised to do the same for creation.
However, the economic value generated by AI isn't distributed evenly. Companies with access to a lot of data will have a huge leg up over those that don't. This could lead to a situation where a handful of companies control most of the economic value created by AI - a handful of infrastructure players and endpoint applications.
Data: The New Coalmine
Just as how the industrial revolution was fuelled by coal, the AI revolution is fuelled by data. And it does voraciously consume data, like a starving puppy at an all-you-can-eat buffet.
Every click, search, and interaction feeds this digital monster, helping fine-tune its algorithms, amp up its predictions, and deliver more tailored experiences. The more data AI has to work with, the sharper its predictions get. This sets off a positive feedback loop: better AI predictions lead to more user engagement, which then generates even more data. This vast reservoir of data has been the bedrock upon which AI models, especially LLMs, have been trained.
However, this symbiotic relationship is not without its frictions. Quality data, once abundant, is becoming a rare commodity, often expensive to collect and curate. As the value of data skyrockets, individuals and institutions are becoming more guarded, wary of sharing their digital footprints. This protective stance, while understandable in an age of privacy concerns, poses challenges for AI's continued growth. This could very well be the most important factor in slowing down the growth of AI.
Harnessing data's immense potential while respecting privacy and navigating the increasing scarcity of quality data will be pivotal in shaping AI's future.
Looking into the economics of AI, it's clear that the factors driving its production and use have a big impact on the competitive landscape. The dropping costs of predictions, the rising value of data, and the changing relationship between creators and consumers aren't happening in isolation.
These economic realities give rise to specific market structures, from dominant oligopolies fuelled by vast data reservoirs to open-source platforms where cooperation and competition coexist. Let's explore how the underlying economics of AI moulds the market landscape, influencing business strategies, competition, and the future trajectory of the industry.
Predictions and Potential Market Dynamics
Given that these are predictions, I will likely be wrong on many of these, and will revisit them at a later date to see how things evolved.
1. Data-Rich Oligopolies: As data becomes increasingly valuable for improving AI predictions, companies with access to vast amounts of high-quality data will likely dominate their respective markets. This could lead to the emergence of data-rich oligopolies, where a few key players control the majority of the market share. What happens to consumer choice and privacy in such a scenario?
2. The Better Distributor will beat the Better Creator: One of my favourite businesses is Appsumo. If you spend a few months there observing all the deals coming through, you can pretty much sense what the current technology wave is. In the past few years, I have seen tens of AI-copywriters, Zapier clones, Podcast tools etc. What really is the difference between one AI-copy writer and its competitor? For all you know, these clones might as well be plugged into OpenAI.
Firms with superior distribution strategies might overshadow those with slightly better products but weaker distribution networks. Since the marginal cost of creation is tending to zero, the winner then, is not the one that has the most features, it's the one which can get the most users. The better distributor wins.
3. Diverse Range of Models: Organisations will likely use a mix of specialised and generalised models, depending on the data availability and bespoke-ness of the task. This will lead to a diverse range of AI applications, from highly specialised models trained from scratch to generalist models fine-tuned or used as-is for tasks with limited data.
For example, in the healthcare sector, specialised AI models are being used for precise diagnostic procedures, while generalised models find applications in patient management systems.
4. Evolution Towards Specialisation: As organisations collect more data over time, they will likely move towards more specialised models for tasks initially tackled with general models. However, the continuous development of new generalised models will also enable new tasks to be accomplished without data, leading to a dynamic and evolving landscape of AI applications. Another byproduct of this evolution - the transition will necessitate a re-evaluation of existing data infrastructures to support the demands of specialised AI applications.
5. Data Generating Use Cases as a Strategic Advantage: Organisations that have use cases generating large amounts of data will have a strategic advantage in the development of fine-tuned AI models. These organisations will be able to continuously improve and specialise their models based on the data generated from their operations.
Imagine the likes of Uber, with millions of rides per day, using their data to fine-tune its AI models for route optimisation, demand prediction, and dynamic pricing. This continuous cycle of data generation, model fine-tuning, and application improvement will create a virtuous cycle that enhances the organisation's competitive advantage over time. Apps generating their own data will be a tremendous investment opportunity as well, since their data-moats will help them defend their market positions really well.
6. Increased Demand for Localised AI Models: With the rise of data privacy regulations and the recognition of cultural nuances, there will likely be an increased demand for localised AI models, leading to the emergence of regional players specialising in culturally and linguistically tailored AI services.
7. Barrier to Entry for Small Players: The increasing importance of data, the high costs associated with AI development, and the competitive advantage of comprehensive platforms may raise barriers to entry for smaller players, making it difficult for them to compete in certain markets. Another significant barrier to entry could be poorly thought out legislation (see Point 10).
8. Open Source Eating Away at Proprietary Models' Market Share: In the open-source world, a special mix of cooperation and competition comes to life. Companies might all chip in to machine learning libraries like TensorFlow, creating a sense of community, while duking it out in the wider AI market, each trying to grab a bigger piece of the pie.
This dynamic puts a lot of pressure on proprietary models to innovate fast and release updates often. OpenAI, for example, felt this pressure when it had to open its doors to users after Midjourney quickly racked up millions of users, while OpenAI's waiting list was capped at just 1,000 signups per week. This incident highlights the competitive challenges faced by proprietary models in a market that's increasingly influenced by open-source initiatives.
9. Geopolitical Influences on Market Structures: The recent export restrictions on certain Nvidia chips to China and some Middle Eastern countries, is likely to foster the emergence of regional powerhouses in these areas, developing independent technological capabilities and localised data-driven oligopolies. This shouldn’t be a surprise - it replicates market structures in many existing internet technologies (Eg, Baidu, Yandex, Naver etc).
10. Poorly Thought Out Legislations: We have seen comical congressional hearings such as those where Mark Zuckerberg had to explain how the internet works, and where Sundar Pichai had to explain that the iPhone was not made by Google. Fundamentally, this is due to career politicians not understanding technology. Carrying on the comical tradition, and on similar lines to Rashida Tlaib’s harebrained stablecoin bill, we now have a bi-partisan AI framework proposed by Senators Blumenthal and Hawley. Amongst the proposals is this:
Establish a Licensing Regime Administered by an Independent Oversight Body. Companies developing sophisticated general purpose AI models (e.g.,GPT-4) or models used in high risk situations (e.g., facial recognition) should be required to register with an independent oversight body, which would have the authority to audit companies seeking licenses and cooperating with other enforcers such as state Attorneys General. The entity should also monitor and report on technological developments and economic impacts of AI.
What, for example, is “sophisticated”? Is facial recognition the threshold for “high risk”? How would an Attorney General who has never written a line of code in his life audit an LLM?
These procedural issues aside, there may very well be a deregulation arms race - the EU and the US can take a lead on regulating any innovation out of AI by imposing substantial financial and operational burdens on emerging companies, perhaps other jurisdictions (such as Dubai, Singapore) could take a more permissive regulatory environment that fosters rapid innovation.
The challenge with regulating AI here is two-fold:
- Pre-defined performance criteria will be outdated soon: Self-explanatory. My very sophisticated gaming computer from the late 90s with 512mb RAM has less RAM than my iPhone, by a factor of over 10. Similarly, the rapid advancement in computing power and open-source community efforts are likely to make it feasible to run sophisticated AI models on personal devices in the near future, potentially decentralising AI capabilities. Smaller teams working on such may not have the resources to go file the paperwork for such licences - can you imagine having to file for a licence to work on an open source project on Github?
- The dilemma of adjusting regulatory thresholds over time, which while allowing focus on companies building the most powerful models, might also leave a gap where unlicensed models can rapidly advance, possibly falling into the hands of malicious actors.
These senators might be well intentioned, but they are spinning vinyls here in the Spotify era.
In Conclusion
We are at the cusp of a Kuhnian revolution, and many of our old ideas about how technology works will just have to be discarded, while some will be retained. The value of technology lies not just in its economic implications, but also as a great leveller. A weak human with a bulldozer could outwork 1000 strong labourers, a novice could beat a grandmaster at chess, and everyday humans can communicate with Presidents and CEOs alike.
The traditional metrics of value are being upended, giving way to a landscape where data is the new currency, and creation costs are minimal. In this evolving narrative, the winners will be those who can navigate the complex interplay of data and innovation, carving out niches that leverage the unique strengths of AI. It is a time of unprecedented opportunity, a chance to redefine the boundaries of what is possible and shape a future that is as dynamic as it is promising.
Member discussion